Chair in Probability Theory
Prof. Dr. Leif Döring
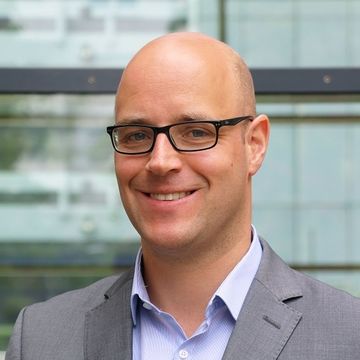
Welcome to the chair of probability theory! Our group has a strong focus in research and teaching on theory and applications in AI of stochastic process theory. The current focus of our research is to use insight from stochastic process theory to improve deep reinforcement learning (RL) algorithms. In reinforcement learning one aims to solve stochastic optimal control problems using only interactions with the problem, but no analytic problem insights. The problem is quite old but was revitalized during the past decade with (mostly) Deepmind's contributions to merge deep learning and RL understanding. While achievements are outstanding the technique is still very inefficient, there is plenty of room for improvement.
On the theory side we work on classical stochasti process topics, including
- Markov process theory
- Stochastic Differential Equations (with jumps)
- Lévy- and Self-Similar Markov Processes
- Branching Processes
- Non-parametric statistics
Stochastic processes are central in the growth of probability theory during the past decades and have various applications in industry for instance in insurance and banking. The importance in AI problems is only starting to get visible in important fields such as diffusion models or reinforcement learing.